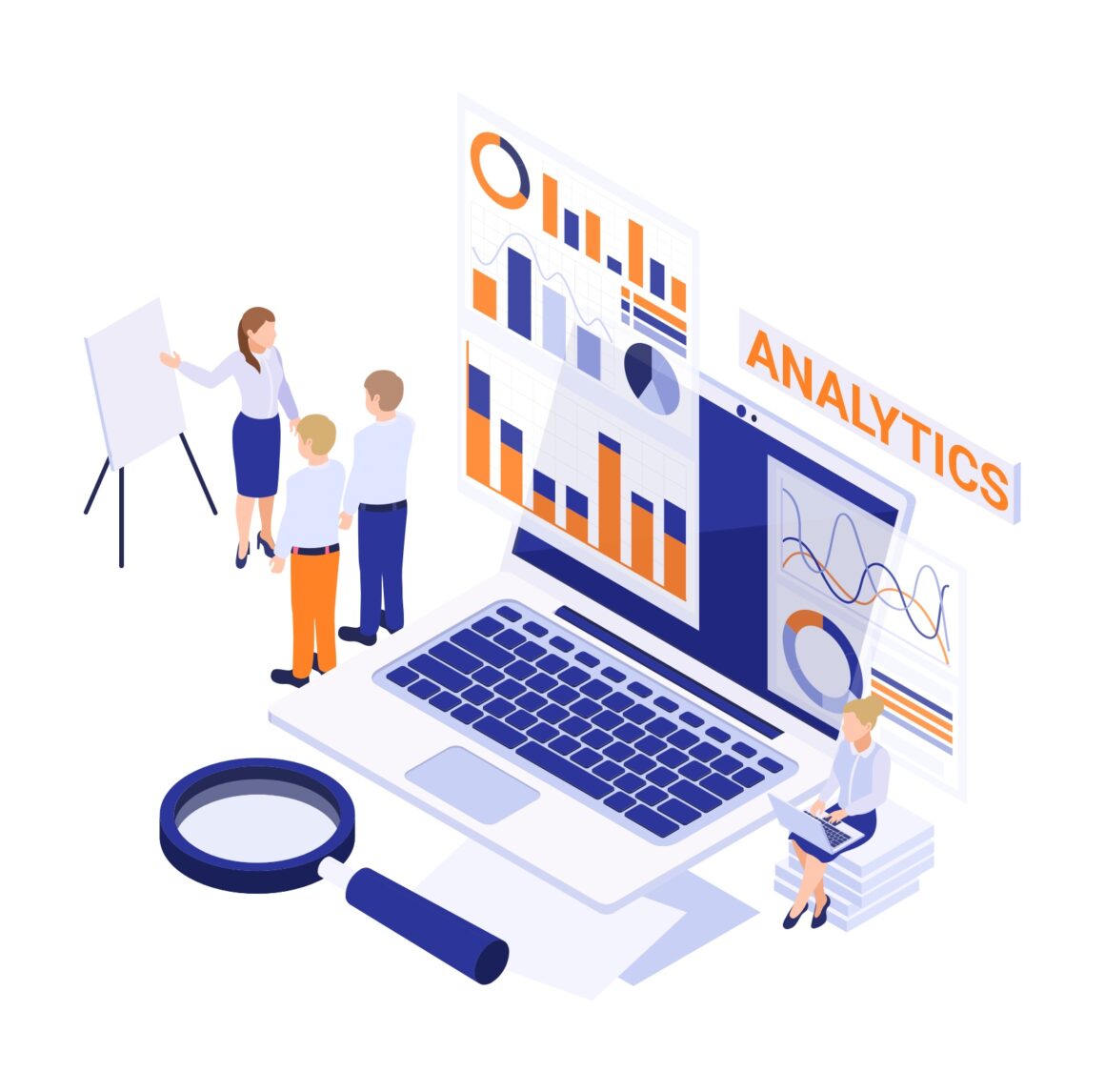
Part of fostering a digital workflow and promoting process automation is improving the quality of the financial data in your business. After running into this step in the process, many CFOs falter because of the variety of data types in use by companies today.
Inconsistency is the enemy of proper automation, so knowing about the types of data out there can greatly improve your chances. Let’s explore the distinction between structured and unstructured data and how they relate to supporting the digital transformation of master data and financial processes.
Understanding structured data
A structured data set is organized, quantifiable, consistently formatted, and easily understood by software and algorithms. This type of data is ideal for process automation since technology, in general, will have no problems parsing, searching, and editing the information.
Examples in finance include:
- Names, phone numbers, and addresses
- Accounting
- Invoice line-items
- Payment information like credit card numbers
- Geolocation data
Even though different formats exist for the same type of structured data (phone numbers might include parentheses, spaces, or just hyphens between the numbers, for instance), converting between formats is easy and still doable by a machine.
You might see structured data used in the accounting department for invoices and receipts whenever transaction dates and monetary amounts are recorded. The customer relationship management (CRM) tool your business uses also deals heavily with structured data sets.
One of the only downsides to structured data is that it’s not as flexible. One format is only useful for the intended purpose it’s designed for.
Understanding unstructured data
Unstructured data is exactly what it sounds like: data that lacks a simple structure that most automated tools and platforms have trouble parsing. Examples include:
- Information in PDF invoices and printed receipts
- Agreements and contracts written in emails and messages
- Social media posts
- Data from electronic sensors and mobile devices
Compared to structured data, this type of information comes in a “raw” format that’s adaptable to any purpose you might need later. Businesses often use it for data mining purposes or the development of chatbots. Without the need for a predefined structure, it’s also much faster to collect and store.
But because it’s difficult to parse and process, using it alongside your automation tools will be a challenge. You need either a data refinement process or a specialized set of tools that can handle unstructured data.
Unstructured data will be a major focus in the coming years, as it makes up nearly 4/5ths of all internal data used by enterprises. Having a data management plan in place that takes into account unstructured points will be a priority.
What about semi-structured data?
Enterprise data is vast and varied, so it should be no surprise that some types of information straddle the line between structured and unstructured. For example, JSON and XML data come with “metadata,” or semantic markers that help identify characteristics of an otherwise unstructured data set.
In other words, semi-structured information is easier to work with despite not being fully structured. Metadata might take the form of headlines or image alt-texts in an online article to help determine what the text is about.
The confusion caused by this distinction
It’s easy to tell where potential problems might lie for companies working with vast amounts of data. Inconsistent formats can cause problems for your robotic process automation efforts, and the manual labor required to fix those issues short-term can introduce human error and inefficiencies.
As a CFO determined to take the next step in the digital transformation of your finance department, it’s your duty to get on top of this confusion before it impacts your business operations. Find a way to convert analog input into usable digital data. Streamline financial processes this way to boost productivity while cutting down on error rates. And overall ensure that you are in tune with the big data analytics market, which is set to reach a value of $103 trillion by 2023.
Are you looking to learn more about how you can support the automation of your financial processes with high-quality, structured data? Download Automation for CFOs today to get access to tips, strategies, and insights.