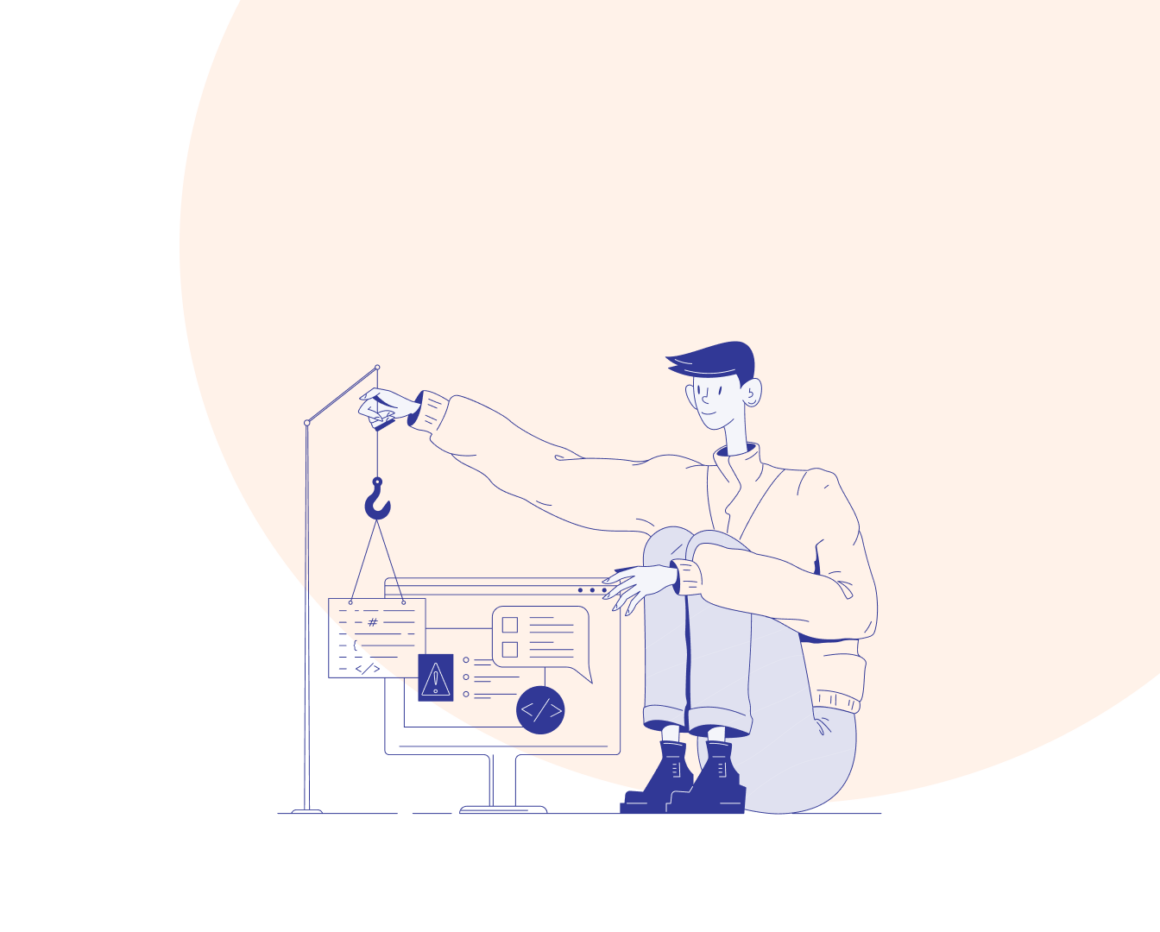
Artificial intelligence (AI) has rapidly become a key focus across industries, especially finance. Financial services and accounting generate large volumes of data, which can be captured, refined, and put to work in a variety of ways.
AI is an umbrella term for several advanced technologies. Understanding these core fields helps financial professionals identify the right tools for their needs and challenges.
This article presents the most relevant AI technologies and techniques driving real impact in finance, so you can determine which are best suited for your business.
AI in finance: technologies and techniques
AI models and techniques have significantly impacted the financial sector, offering sophisticated tools for analyzing transactional data, master data, accounting records, etc. Here are some AI techniques commonly used in financial data analysis:
Large language models (LLMs)
Advanced AI models trained on massive text datasets. LLMs excel at generating, summarizing, and interpreting natural language, making them ideal for tasks like automating financial reporting, analyzing contracts, answering compliance questions, and enhancing customer communication. LLMs are technically a subset of deep learning and are often considered part of natural language processing (NLP).
Machine learning (ML) models
These include supervised learning models like regression analysis (linear and logistic regression) for predicting future trends based on past data and unsupervised learning models like clustering (K-means, hierarchical clustering) for segmenting data into meaningful groups.
Deep learning models
Neural networks, especially deep neural networks (DNNs), convolutional neural networks (CNNs), and recurrent neural networks (RNNs), are powerful for complex pattern recognition in data, making them suitable for fraud detection, customer behavior analysis, and predictive analytics.
Natural language processing (NLP)
Used for analyzing financial documents, reports, and news to extract valuable insights, sentiment analysis, or to automate customer service and advisory services.
Decision trees and random forests
As supervised ML models, decision trees and random forests are helpful for classification and regression tasks, such as credit scoring, risk assessment, and customer segmentation.
Time series analysis models
Models like ARIMA (Autoregressive Integrated Moving Average) are used for forecasting financial metrics and analyzing temporal patterns in financial markets.
Reinforcement learning
Applied in algorithmic trading to develop strategies that maximize rewards based on historical data.
Anomaly detection models
Not a model type in itself but rather an application, anomaly detection can be utilized to identify outliers or unusual patterns in financial transactions, which could indicate fraud or errors in the data.
Graph-based models
Effective in detecting complex relationships and networks, such as uncovering fraudulent activities through analysis of transaction networks.
Synthetic data generation
While LLMs handle text, generative AI can also be applied to other data types like synthetic data generation, scenario modeling, and report automation. In finance, this can be useful for simulating economic scenarios for risk management, generating synthetic but realistic transaction data for testing models, and creating dynamic financial forecasts and reports.
These AI techniques can be tailored to specific financial tasks, from automating accounting processes and optimizing financial strategies to enhancing fraud detection mechanisms and improving customer experiences.
The choice of technology, model, and how you use it depends on a multitude of factors, including specific application in finance and accounting, data availability, and the desired accuracy of the outcomes.
Data is the most valuable asset in AI-driven transformation for financial processes. Refining current data management practices prepares businesses to adopt the next generation of tools. To stay competitive, it’s essential for businesses to prioritize converting unstructured data to structured data, establishing an infrastructure for exchange, implementing organization-wide standards, and prioritizing digital real-time processes.
For further reading and practical guidance, download our whitepaper AI for finance teams or explore Qvalia’s AI solutions for finance processes.